
What does ROC AUC mean? AUC - ROC curve is a performance measurement for classification problem at various thresholds settings. ROC is a probability curve and AUC represents degree or measure of separability. It tells how much model is capable of distinguishing between classes.
The Receiver Operator Characteristic ( ROC ) curve is an evaluation metric for binary classification problems. It is a probability curve that plots the TPR against FPR at various threshold values and essentially separates the ‘signal’ from the ‘noise’. A receiver operating characteristic curve , or ROC curve, is a graphical plot that illustrates the diagnostic ability of a binary classifier system as its discrimination threshold is varied.
The ROC curve is created by plotting the true positive rate (TPR) against the false positive rate (FPR) at various threshold settings. AUC – ROC curve is a performance measurement for the classification problems at various threshold settings. The first one is here about the most loved evaluation metric — The ROC curve. ROC ( Receiver Operating Characteristic ) Curve is a way to visualize the performance of a binary classifier.
AUC : Area Under the ROC Curve. AUC stands for Area under the ROC Curve. That is, AUC measures the entire two-dimensional area underneath the entire ROC curve (think integral calculus) from (0) to (1). AUC (Area under the ROC Curve). AUC provides an aggregate measure of performance across all possible classification thresholds.
AUC gives the rate of successful classification by the logistic model. The AUC makes it easy to compare the ROC curve of one model to another. The AUC for the red ROC curve is greater than the AUC for the blue RO C curve. If two ROCs cross, the ROC with the higher AUC will have at least a measurable subset of thresholds where ROC with inferior AUC is a better classifier.
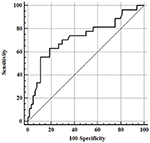
So if I have data points of class and of class and my classifier always predicts class i would still have a accuracy of. It is naturally inherited from any call to roc and fits most cases. A rough guide for classifying the accuracy of a diagnostic test is the traditional academic point system:. In this case, it means the Area Under the ROC Curve ( AUC-ROC ). It is a metric that tells you how separable your positive and negative responses are from each other. This metric varies with the model we choose for the problem in hand.
AUC — ROC curve is a performance measurement for classification problem at various thresholds settings. An ROC curve is generated by plotting the false positive rate of a model against its true positive rate, for each possible cutoff value. Often, the area under the curve ( AUC ) is calculated and used as a metric showing how well a model can. Now we can try our AUC functions on both sets to check that they can handle both step functions and segments with intermediate slopes. If a classifier obtain an AUC -PR smaller than 0. Binary classification tasks are the bread and butter of machine learning.

Here, a performance measure is introduced that simply considers the probability of making a correct binary classification. Warning: if the roc object passed to plot. TRUE, auc is not called and arguments such as partial.
References Xavier Robin, Natacha Turck, Alexandre Hainar et al. ROC curve analysis in MedCalc includes calculation of area under the curve ( AUC ), Youden index, optimal criterion and predictive values. The program generates a full listing of criterion values and coordinates of the ROC curve.
No comments:
Post a Comment
Note: only a member of this blog may post a comment.