Classifiers that give curves closer to the top-left corner indicate a better performance. As a baseline, a random classifier is expected to give points lying along the diagonal (FPR = TPR). Its name is indeed strange.
The larger the AUC, the better is overall performance of the medical test to correctly identify diseased and non-diseased subjects. It tells how much model is capable of distinguishing between classes. By analogy, Higher the AUC, better the model is at distinguishing between patients with disease and no disease.
A rough guide for classifying the accuracy of a diagnostic test is the traditional academic point system:. Summary measures for a desired (user -specified) list of cutoff values are also available. True Positive Rate (TPR) or sensitivity: the proportion of actual positives that are correctly identified as such.
ROC Curves Interpretation. A model with low sensitivity and low specificity will have a curve that is close to the 45-degree diagonal line. The diagnostic test is able to dicriminate if the curve differs significantly from the diagonal (bottom left - top right). In the ideal case (1 selectivity), the curve lies on the left or upper boundary side of the surrounding square.
This procedure is a useful way to evaluate the performance of classificationschemes in which there is one variable with two categories by whichsubjects are classified. This area is a measure of the predictive accuracy of a model. Read more in the User Guide. The idea is to maximize correct classification or detection while minimizing false positives. Receiver operating characteristic (ROC) curves are used in medicine to determine a cutoff value for a clinical test.
For example, the cutoff value of 4. PSA) test for prostate cancer. The points are connected to form the curve. Cutoff values that result in low false-positive rates tend to result low true-positive rates as well. If the vector contains more than two unique values, or if their order could be ambiguous, use levels to specify which values must be used as control and case value.
Signal detection theory is a theory in mathematics, statistics and psychology primarily concerned with the descriptive and normative theories of discriminating between signals and noise. In fact the area under the curve (AUC) can be used for this purpose. It is a plot of the false positive rate (x-axis) versus the true positive rate (y-axis) for a number of different candidate threshold values between 0. For example, the cutoff value of 4. PSA) test for prostate cancer. A test value below 4. This utility calculates test sensitivity and specificity for a test producing a continuous outcome.
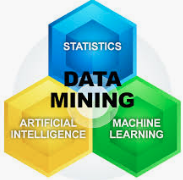
Suggested cut-points are calculated for a range of target values for sensitivity and specificity. The different points on the curve correspond to the different cutpoints used to determine whether the test are positive. Introduction A receiver operating characteristics (ROC) graph is a technique for visualizing, organizing and selecting classifi- ers based on their performance.
The terminology for the inputs is a bit eclectic, but once you figure that out the roc.
No comments:
Post a Comment
Note: only a member of this blog may post a comment.